Selected Projects
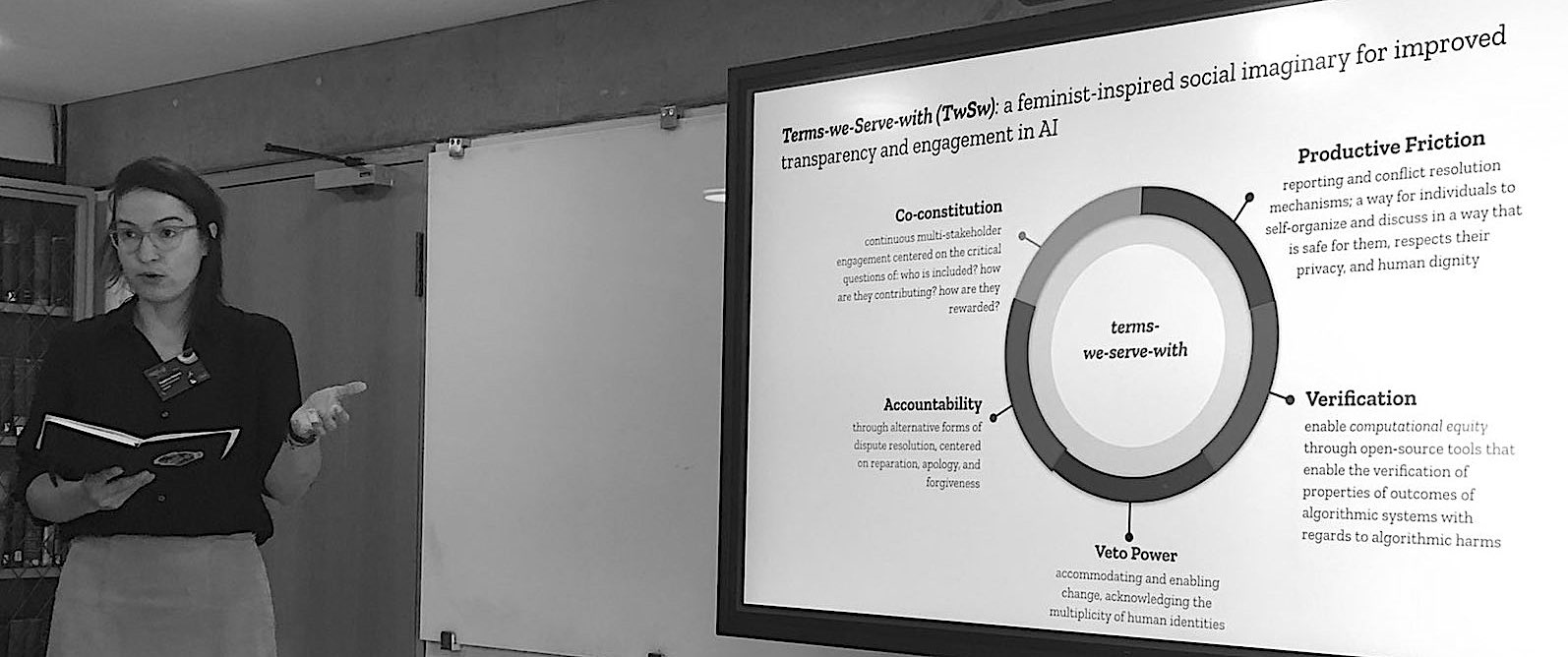
There's a lack of sufficient transparency with regards to the real world impact of ML models. The power and information asymmetries between people and consumer technology companies employing algorithmic systems are legitimized through contractual agreements which have failed to provide people with meaningful consent and contestability, in cases of experiences of algorithmic harms.
- Improved understanding of the challenges that exist in current approaches to transparency of consent and dispute resolution mechanisms put in place by tech companies.
- Evaluation of computable contracts as a transparent socio-technical intervention that builds agency in consumer use of AI.
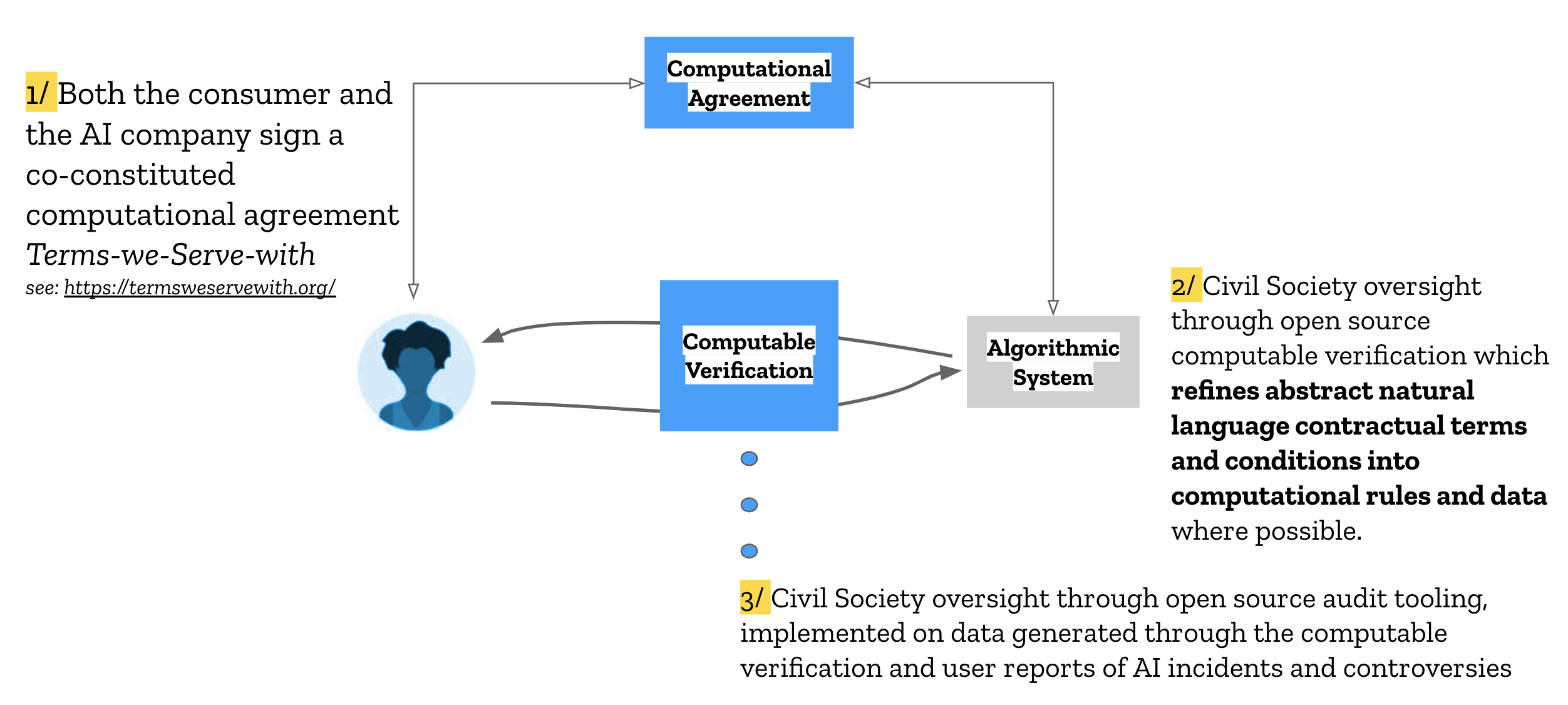
A feminist-inspired social, computational, and legal contract for restructuring power asymmetries and center-periphery dynamics to enable improved human agency in individual and collective experiences of algorithmic harms. It is a provocation and a speculative imaginary centered on the notions of co-constitution, through participatory mechanisms; accountability, through reparation, apology, and forgiveness; positive friction through enabling meaningful dialogue in the production and resolution of conflict; verification through open-sourced computational tools; and veto-power reflecting the temporal dynamics of how individual and collective experiences of algorithmic harm unfold. Read our paper and find out more at: https://termsweservewith.org/
An analysis of the CORD-19 research dataset which aims to investigate the ethical and
social science considerations regarding pandemic outbreak response efforts, also recognized
by Kaggle as the winning submission in the corresponding task of the CORD-19 Kaggle Challenge.
Read more here and play with the source code python notebook yourself.
The diagram shows the number of documents discussing barriers and enablers (blue) vs
implications (red) of pandemic crisis response efforts, relative to specific policy response efforts.
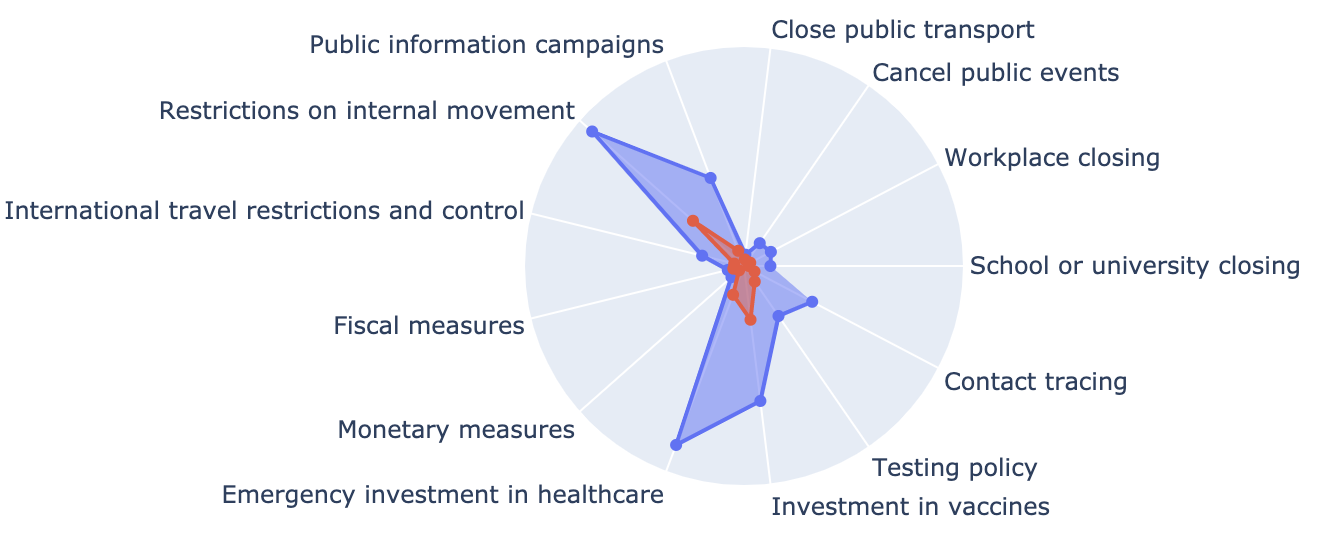
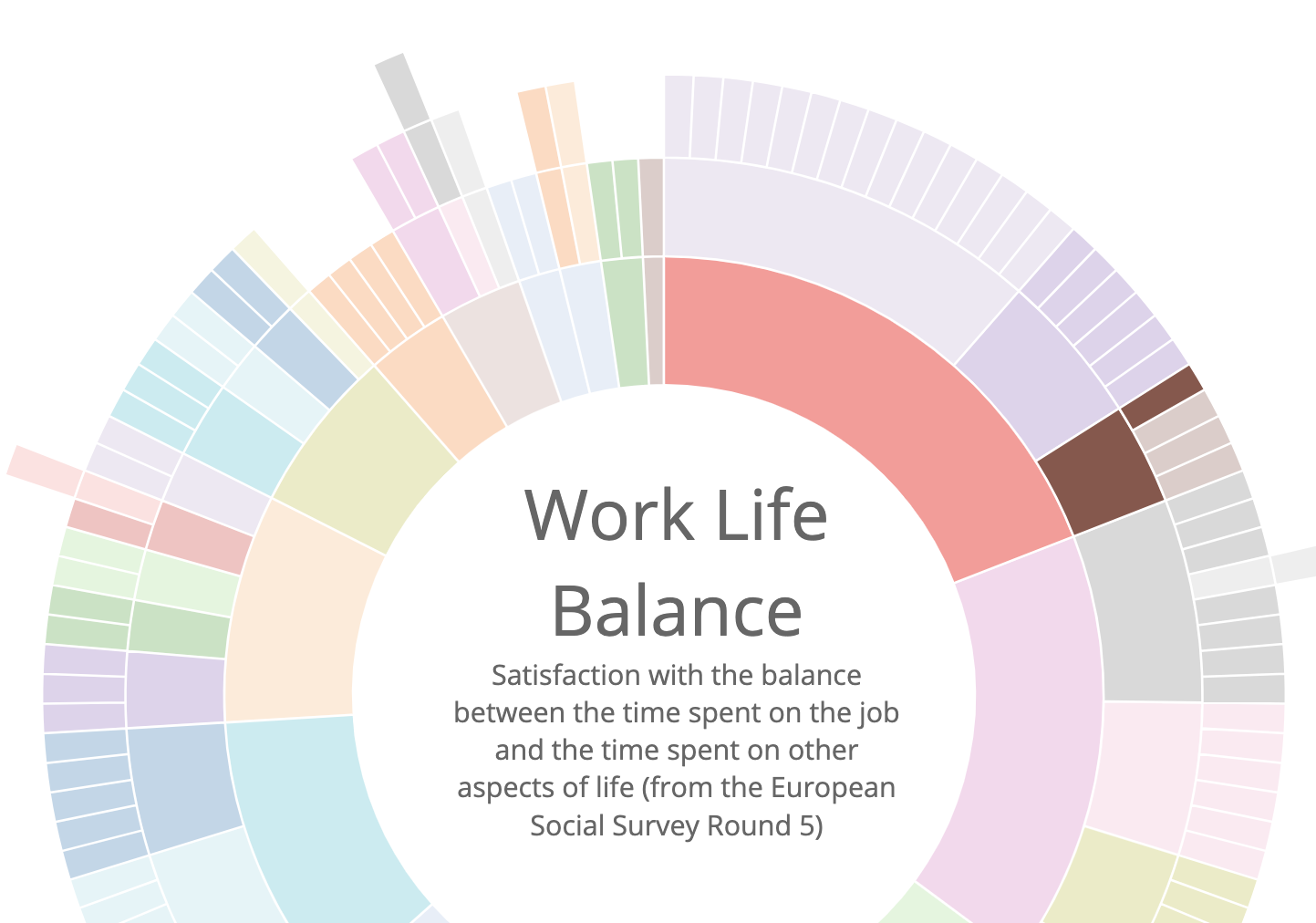
I've been part of the core team at the IEEE P7010 working group developing a recommended practice
for Assessing the Impact of Autonomous and Intelligent Systems on Human Well-Being. Incorporating well-being factors throughout the lifecycle of
AI is both challenging and urgent and IEEE 7010 provides key guidance for those who design, deploy, and procure these technologies.
Read an overview paper introducing the standard here.
I'm also the lead guest editor of an upcoming special issue of the Springer International Journal of Community Well-being focused on the intersections of AI and
community well-being. The special issue explores three topic areas including well-being metrics framework for AI, how can AI protect community well-being from threats, as well as how can AI itself be a threat to communities and what can communities do to mitigate, manage, or negate such threats.
Bringing awareness to our inherent positionality, we gain new perspectives about the relational nature of (un)intended consequences of AI systems. Giving examples from a recent ethnographic study in the intersection of organizational structure and the work on ensuring the responsible development and use of AI, we investigate the so-called socio-technical context - the lived experience of some of the people actively involved in the AI ethics field. Learning from the field of Participatory Relational Design, we investigate what can we learn from the design of social movements (specifically in the Global South) about the way we work to ensure better alignment between AI systems and human and ecological well-being.
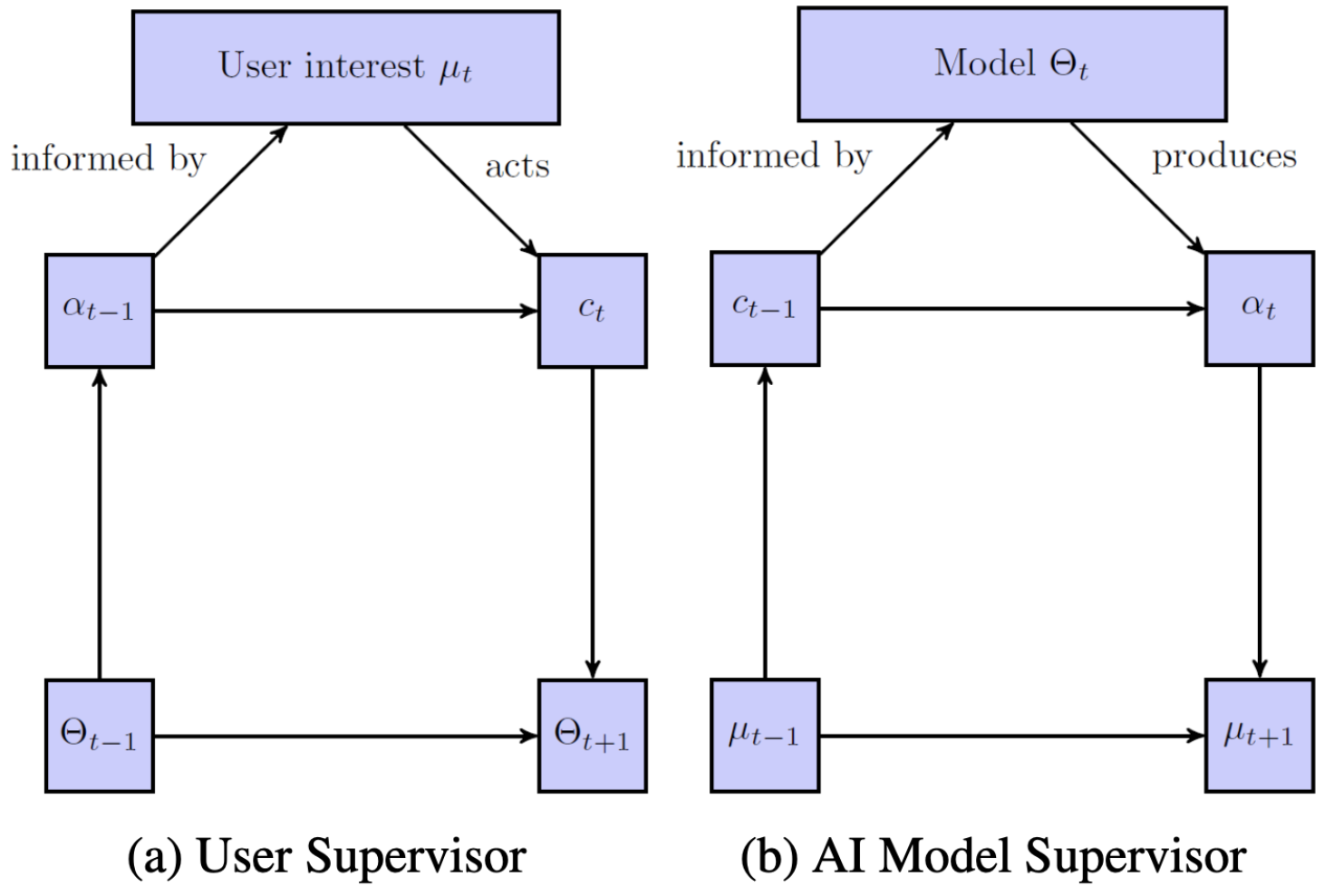
This research paper explored the dynamics of the repeated interactions between a user and a recommender system, leveraging the human-in-the-loop system design from the field of Human Factors and Ergonomics. We go on to derive a human-algorithmic interaction metric called a barrier-to-exit which aims to be a proxy for quantifying the ability of the AI model to recognize and allow for change in user preferences.
A talk I gave at All Tech Is Human event in San Francisco: "Borrowing frames from other fields to think about algorithmic response-ability". See it in written form here.
This tutorial session at the ACM FAT* Conference explored the intersection of organizational structure and responsible AI initiatives and teams within organizations developing or utilizing AI.