Research and Publications
Google ScholarOverlaying themes in Environmental Justice and social-ecological-technological systems analysis, we propose a qualitative framework of questions which could be implemented as part of an iterative algorithmic audit process.
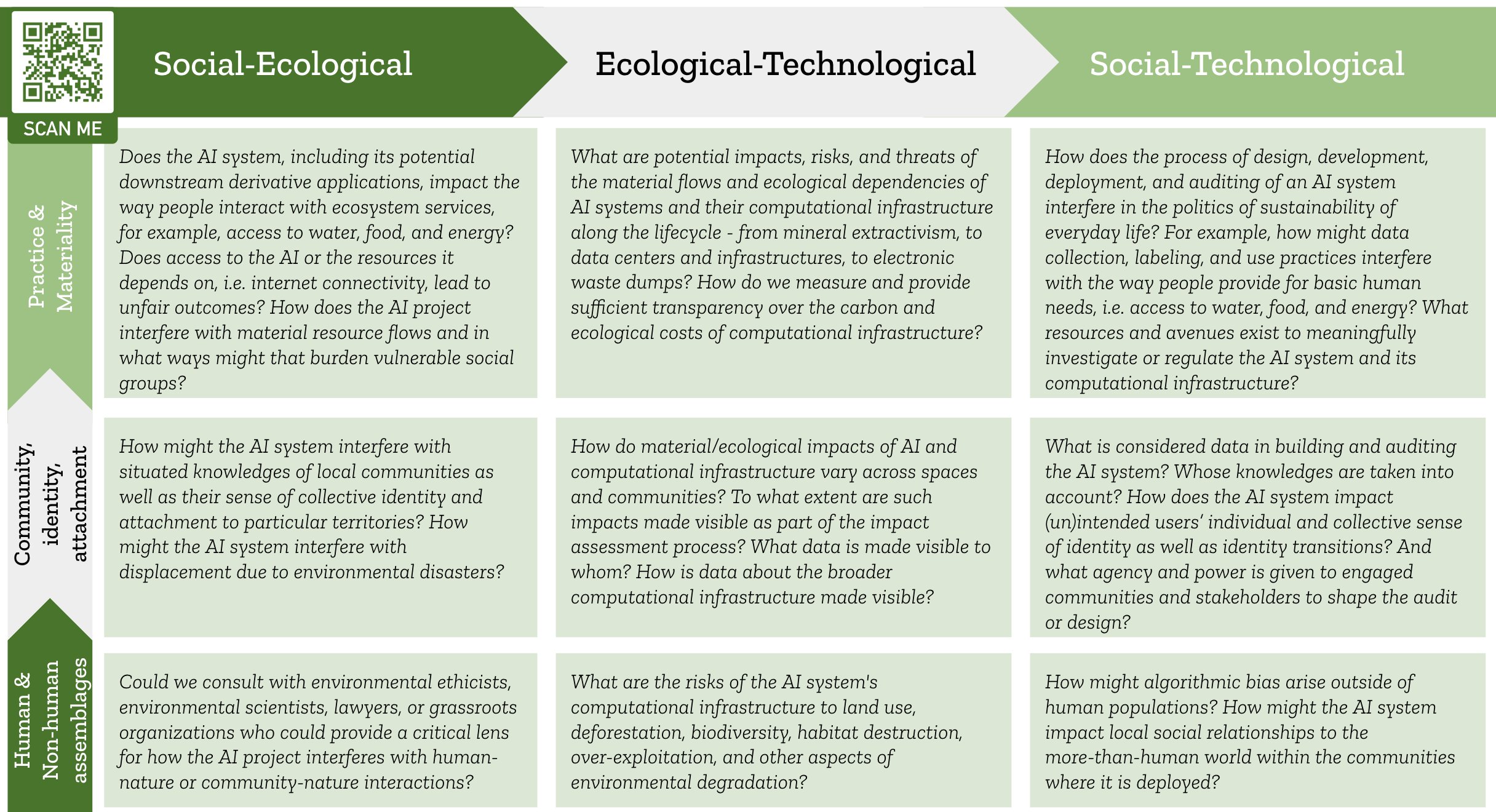
[see the conference talk recording]
This paper examines and seeks to offer a framework for analyzing how organizational culture and structure impact the effectiveness of responsible AI initiatives in practice. We present the results of semi-structured qualitative interviews with practitioners working in industry.
We found that most commonly,
practitioners have to grapple with lack of accountability, ill-informed performance trade-offs and
misalignment of incentives within decision-making structures that are only reactive to external
pressure. Emerging practices that are not yet widespread include the use of organization-level
frameworks and metrics, structural support, and proactive evaluation and mitigation of issues as
they arise. For the future, interviewees aspired to have organizations invest in anticipating and
avoiding harms from their products, redefine results to include societal impact, integrate responsible AI practices throughout all parts of the organization, and align decision-making at all levels
with an organization’s mission and values.
We aim to map the consensus between AI ethics principles and well-being indicators and point to examples on how they could be addressed by well-being indicator frameworks. Leveraging the toolset of policy impact analysis together with well-being indicators could help AI developers and users by providing ex-ante and and ex-post means for the analysis of the AI impacts on well-being. As discussed by Daniel Schiff et al., the well-being impact assessment involves the iterative process of (1) internal analysis, informed by user and stakeholder engagement, (2) development and refinement of a well-being indicator dashboard, (3) data planning and collection, (4) data analysis of the evaluation outputs that could inform improvements for the A/IS.
Building on work in Policy, we aim to explore existing governance frameworks in the context of Sustainability. Prof Thomas Hale at the Blavatnik School of Government, Univ of Oxford, has written about the concept of catalytic cooperation, catalytic effects, and ultimately the potential and benefits from catalytic institutions in the context of Climate Action. According to his model, increasing the number of actors involved in forest regeneration efforts lowers the costs and risks for more actors to become involved in this space until the kickstart of a “catalytic effect”, which could lead to cooperation over time. By doing a comparative analysis of the problem structures of reducing environmental degradation and reducing the negative impacts of AI, we find that they exhibit similarities in their distributional effects, the spread of individual vs. collective harms, and first-order vs. second order impacts. Hence, we propose that it is helpful to restructure and address AI governance questions through a catalytic cooperation model.
We discuss five sociotechnical dimensions characterizing the gap in the interface layer between people and AI - accommodating and enabling change, co-constitution, reflective directionality, friction, and generativity. Reinforcing the need to go beyond accuracy metrics, academic researchers and practitioners have a responsibility to investigate and spread awareness about the (un)intended consequences of the AI algorithms and systems to which they contribute. Ultimately, new kinds of metrics frameworks, behavioral licencing or ToS agreements could empower participation and inclusion in the responsible development and use of AI.